Unveiling the Dark Side of AI: How Artificial Intelligence Goes Wrong with Misperceived Realities
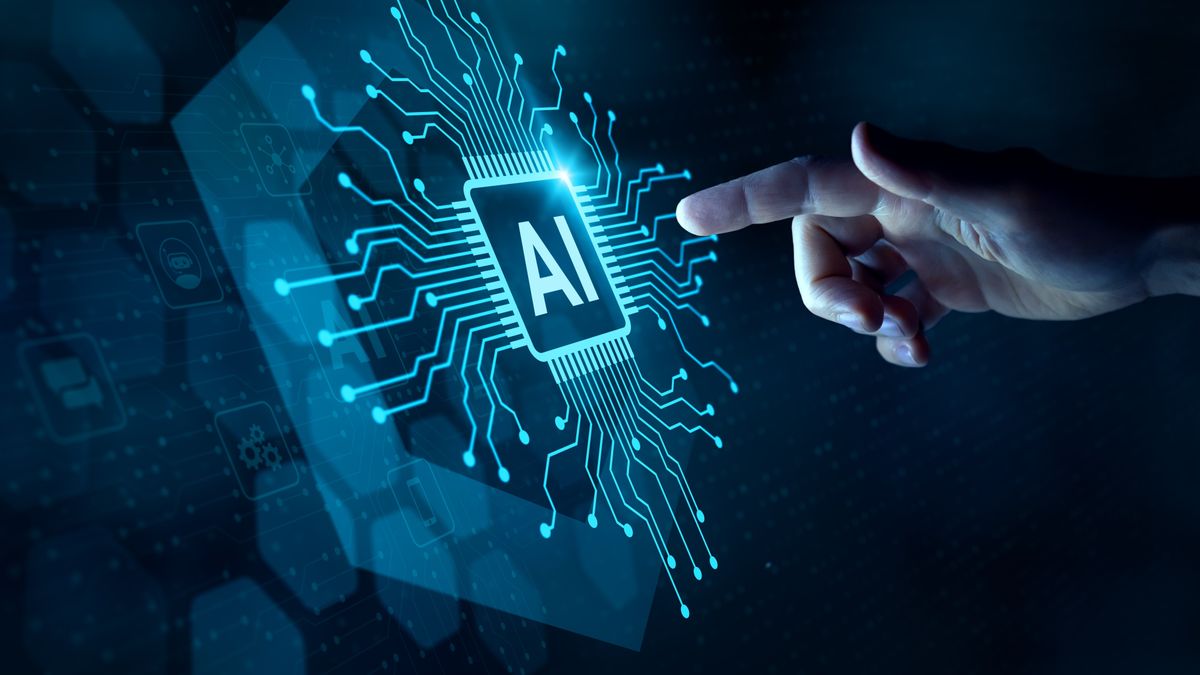
The Dark Side of AI: Understanding and Preventing Hallucinations
Imagine receiving an answer to a question from a trusted AI tool, only to discover it’s entirely fabricated or inaccurate. This phenomenon is known as AI hallucination, where generative AI tools respond to queries with false information. In this article, we’ll delve into the world of AI hallucinations, explore the reasons behind this issue, and discuss how to prevent it.
What are AI Hallucinations?
AI hallucinations are instances where a generative AI tool responds to a query with statements that are factually incorrect, irrelevant, or entirely fabricated. For example, Google’s Bard falsely claimed that the James Webb Space Telescope had captured the first-ever pictures of a planet outside our solar system. Similarly, two New York lawyers were sanctioned by a judge for citing six fictitious cases in their submissions, which were prepared with the assistance of ChatGPT.
Why does AI hallucinate?
Generative AI models work by predicting the next most likely word or phrase from what they’ve seen. If they don’t understand the data they’re being fed, they’ll produce something that might sound reasonable but isn’t factually correct. According to Simona Vasytë, CEO at Perfection42, AI looks at the surroundings and "guesses" which pixel to put in place. Sometimes, it guesses incorrectly, resulting in a hallucination.
Consequences of AI Hallucinations
When AI hallucinations occur, the consequences can be severe. As two New York lawyers discovered, AI hallucinations aren’t just an annoyance. They can lead to costly mistakes in critical areas like law and finance. Experts believe it’s essential to eliminate hallucinations to maintain faith in AI systems and ensure they deliver reliable results.
Why is it important to eliminate hallucinations?
AI hallucinations can lead to myriad problems, including costly mistakes and damages. According to Olga Beregovaya, VP of AI and Machine Translation at Smartling, hallucinations will create as many liability issues as the content the model generates or translates. She suggests legal contracts, litigation case conclusions, or medical advice should always go through human validation.
Eliminating Hallucinations
Experts say reducing hallucinations is achievable, and it all begins with the datasets the models are trained on. Vasytë asserts high-quality, factual datasets will result in fewer hallucinations. She suggests companies that invest in their own AI models will achieve solutions with the least AI hallucinations. Curtis agrees, but suggests companies should use a representative dataset that’s been carefully annotated and labeled.
Hallucination-Free AI
While eliminating AI hallucinations is a challenging task, reducing them is certainly feasible. Some experts propose using retrieval augmented generation (RAG) for addressing the hallucination problem. Instead of using everything it was trained on, RAG gives generative AI tools a mechanism to filter down to only relevant data to generate a response. This results in outputs that are more accurate and trustworthy.
Conclusion
AI hallucinations are a growing concern in the world of artificial intelligence. As we continue to rely on AI tools to make decisions, it’s essential to understand the risks associated with AI hallucinations. By recognizing the root causes of this phenomenon and implementing measures to prevent it, we can ensure that AI systems deliver reliable and accurate results. Stay tuned for more updates on this crucial topic.